Understanding consumer behavior and trends is necessary for businesses aiming to stay competitive. Predictive analytics offers powerful tools to forecast these behaviors and trends accurately. By anticipating consumer actions, companies can make proactive decisions, enhance their strategies, and drive growth. With the rapid advancements in data analytics and machine learning, businesses can now delve deeper into consumer data, uncovering previously inaccessible insights. This comprehensive guide will provide a detailed look at how predictive analytics is helping businesses make informed decisions and stay ahead in the market.
Understanding Consumer Behavior and Trends
Consumer behavior refers to individuals’ actions and decision-making processes when purchasing goods or services. It encompasses various factors like psychological, social, and economic influences. Consumer trends are the patterns and shifts in consumer behavior over time. Accurately predicting these behaviors and trends helps businesses tailor their strategies, optimize inventory, and enhance customer satisfaction.
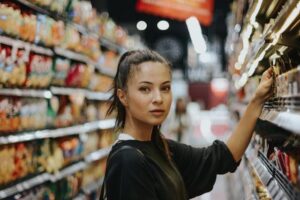
What is Predictive Analytics?
Predictive analytics involves using historical data, statistical algorithms, and machine learning techniques to identify the likelihood of future outcomes. By analyzing past data, predictive models can forecast future behaviors and trends. This process enables businesses to make proactive decisions, enhancing their ability to meet consumer needs effectively. Predictive analytics can be applied across various domains, including marketing, finance, supply chain, and customer service. By leveraging these advanced techniques, businesses can gain a competitive edge, improve operational efficiency, and create more personalized customer experiences, leading to increased loyalty and higher revenue.
Using Data Analytics to Understand User Behavior
Data analytics tools provide invaluable insights into user behavior across digital platforms. Businesses can uncover patterns that illuminate how users interact with their online presence by analyzing metrics such as page views, session durations, and conversion rates. This understanding allows companies to optimize user experiences by identifying popular content, improving navigation flow, and enhancing overall site usability. For instance, identifying pages with high bounce rates can guide strategies to improve content relevance or page load times, thereby reducing bounce rates and increasing engagement.
Advanced analytics tools, like Google Analytics, offer features such as cohort analysis and user journey mapping, enabling businesses to segment users based on behavior and track their interactions over time. Once you learn more about user behavior using Google Analytics, you can optimize your website and digital marketing strategies to meet your audience’s needs and preferences better.
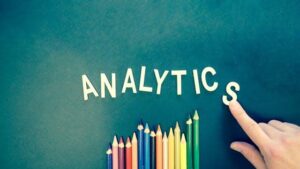
Key Techniques in Predictive Analytics
Predictive analytics employs several techniques to forecast consumer behavior and trends. Statistical methods like regression analysis and time series analysis are commonly used. Machine learning methods provide more advanced forecasting capabilities, including clustering, classification, and neural networks. For instance, clustering can segment consumers into groups with similar behaviors, while neural networks can detect complex patterns in large datasets. These techniques enable businesses to uncover hidden insights, predict future behaviors more accurately, and develop strategies tailored to specific consumer segments.
How to Use Predictive Analytics
1. Data Collection
The first step is identifying relevant data sources. That can include internal sources like sales records and external sources like market research. Collecting high-quality data involves accuracy, consistency, and relevance. Businesses should also consider data privacy and ethical implications when gathering consumer information. Effective data collection practices lay the foundation for accurate predictive models, enabling businesses to capture a comprehensive view of consumer behavior.
2. Data Preparation
Once data is collected, it needs to be cleaned and preprocessed. That involves handling missing values, correcting inconsistencies, and normalizing data. Proper data preparation will help build robust predictive models. Tools like data wrangling software can automate much of this process, saving time and reducing errors. Correctly preparing data ensures that predictive models are based on reliable and accurate information, enhancing their predictive power.
3. Model Selection
Choosing the right predictive model is critical. Thus, businesses should compare different models to find the best fit for their data and objectives. Common models include linear regression for simple trends and more complex algorithms like decision trees and neural networks for intricate patterns. Model selection often involves a trade-off between simplicity and accuracy. The right model should balance these factors to provide reliable predictions without unnecessary complexity.
4. Model Training and Testing
Training the model involves using historical data to teach the algorithm about past patterns. Then, you must test the model on a separate dataset to evaluate its accuracy. Cross-validation techniques can help make sure the model generalizes well to new data. Continuous testing and validation are necessary to maintain model performance over time.
5. Forecasting
With a trained model, businesses can start making predictions. These forecasts can inform various business decisions, from inventory management to marketing strategies. Interpreting the results involves understanding the model’s outputs and implications for business operations. Likewise, visualization tools can help make these predictions more accessible and actionable. This step is necessary to translate predictive analytics into tangible business outcomes aligning with organizational goals.
Future Trends in Predictive Analytics for Consumer Behavior
Advances in AI and machine learning are enhancing predictive analytics capabilities. Integration with technologies like IoT and blockchain is expanding the scope of data available for analysis. These innovations pave the way for more accurate and granular forecasts, helping businesses stay ahead in an increasingly dynamic market. These technologies will enable even more sophisticated analyses and deeper insights as they evolve. Therefore, businesses that embrace these future trends will be well-positioned to anticipate and respond to changes in consumer behavior, maintaining a competitive advantage.
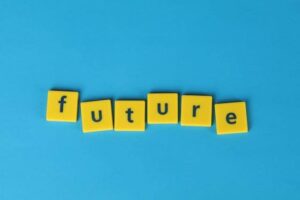
Conclusion
In conclusion, forecasting consumer behavior and trends using predictive analytics is a powerful approach for businesses aiming to thrive in today’s competitive landscape. By leveraging historical data and advanced algorithms, companies can make informed decisions, optimize operations, and employ digital marketing. Companies that effectively utilize predictive analytics will be better equipped to meet the evolving needs of their customers and achieve long-term success.